Most of the readers of this blog would be familiar with ordinary least squares estimator and regression models. Let us talk about one source that can cause these estimates and models to be biased and inconsistent. This is especially important when we think about the causal relationship of interest or the relationship which being studied. Proving causality can be difficult. But if we assess our econometric analysis and consider the different threats to the validity of our statistical inference then we can be more assured about our analysis, results, and inferences.
We shall be discussing omitted variables bias. This is bias that occurs when
- the regressor X is correlated with an omitted variable Z.
- omitted variable Z is a determinant of the dependent variable Y.
Both of these conditions result in the violation of the Gauss-Markov assumption of ordinary least squares regression
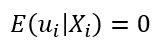
which is the assumption that states that the error term is uncorrelated with the regressors. The u denotes the error term while the X denotes the regressors. Simply put, this bias occurs when an econometric model leaves out one or more relevant variables. This bias results from the model attributing the effects of the missing variable(s) to those that are included in the model.
One simple example of this bias is by taking an example of dependent variable Salary, regressor Education and omitted variable Ability. Here, Salary is the annual salary of the individuals in our sample. Education can be either years of education or their scores on tests or any other measure of education. Ability is some variable which signifies talent, skill, or proficiency in general. We can think of Ability as being unmeasurable as well.
Let us think about a bias induced when we omit the Ability variable as a regressor, either due to a mistake or because we cannot measure it. At the same time, the true data generating process includes Ability and is

In this case, the variable Ability would have some impact on both Salary as well as Education. Hence, we can say that Ability is correlated with Education. The effect on Salary could be directly captured by including it in the regression. At the same time, we have no method to quantify Ability. And if we do not include it then its effect on Salary will not be picked up as an indirect effect through the variable Education.
If we do not use the true data generating process and instead use

Then we run into the problem of omitted variable bias. This causes our ordinary least squares estimate of the estimate of Education (denoted by beta_1)to be biased and inconsistent. This means that the bias cannot be prevented by increasing the sample size because omitted variable bias prevents ordinary least squares estimate from converging in probability to the true parameter value. The strength and direction of the bias is determined by the correlation between the error term and the regressor.
Now that we know exactly what the issue of Omitted Variable Bias is, let us consider some solutions.
One answer to this issue is to include more variables in the regression model. By doing this, the regression model uses as independent variables, not only the ones whose effects on the dependent variable are of interest, but also any potential variables which might cause omitted variables bias. Including these additional variables can help us reduce the risk of inducing omitted variables bias but at the same time, it may increase the variance of the estimator.
Some general guidelines to follow in this case that help us in our decision to include additional variables are:
- Specify the coefficient of interest.
- Based on your knowledge of the variables and model, identify possible sources of omitted variables bias. This should give you a starting point specification as baseline and a set of regressor variables, sometimes called control variables.
- Use different model specifications and test against your baseline.
- Use tables to provide full disclosure of your results – by presenting different model specifications, you can support your argument and enable readers to see the impact of including other regressors.
If diminishing the bias by including additional variables is not possible, such as in the cases where there are no adequate control variables, then there are still a variety of approaches which can help us solve this problem.
- Making use of panel data methods.
- Making use of instrumental variables regression methods such as Two Stage Least Squares.
- Making use of a randomized control experiment.
These approaches are important to consider because they help us to avoid false inferences of causality due to the presence of another underlying variable, the omitted variable, that influences both the independent and dependent variables.
Bibliography:
Wooldridge, Jeffrey M. (2009). “Omitted Variable Bias: The Simple Case”. Introductory Econometrics: A Modern Approach. Mason, OH: Cengage Learning. ISBN 9780324660548.
Greene, W. H. (1993). Econometric Analysis (2nd ed.). Macmillan.